Hedge Funds Hunt for Alpha Across 1 Billion Smartphones
Mobile geolocation has opened a new frontier for investors
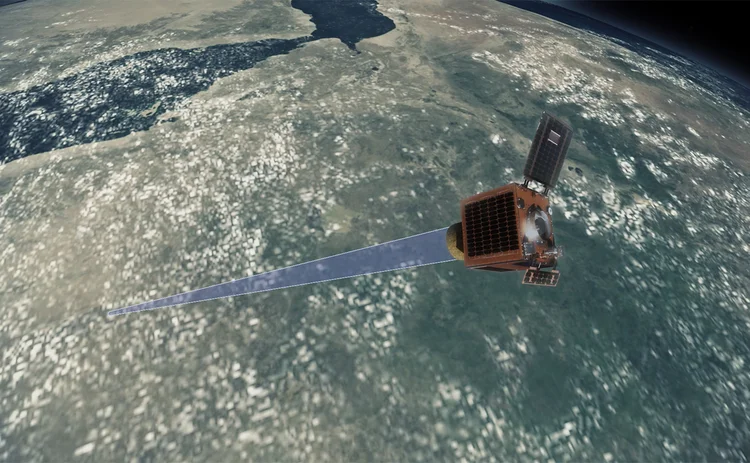
Need to know
- The most common way geolocational data is collected is by asking consumers for their location through mobile applications.
- Buying geolocational data is not enough. Hedge funds need staff with the proper skills to generate alpha from it.
- Mobile device users are explicitly informed their information will be used and resold, making geolocation arguably more transparent then other data sets.
- The growth of 5G networks will improve accuracy, but lack of historical data means investors will have to wait a year or two to get results.
In 2011, John Collins was a graduate student at the Massachusetts Institute of Technology (MIT), focused on finance and statistics. His life changed during class one day, when he met two people who shared common interests about leveraging non-financial data sources for drawing economic insights. One was Greg Skibiski, a visiting lecturer, and the other, Wei Pan, a PhD student in computational science.
One development led to another, and the next year, all three found themselves working together at Thasos Group, a company that had been incorporated with Skibiski as its CEO and chairman. They were based across the street from the college, and heavily leveraged connections across the campus in the early stages of the company. “All of our early hires were from MIT,” he recalls.
From 2013 onward, they ran a portfolio that was data-driven in terms of investment decisions. By 2015 they were managing $100 million. They were also interfacing with other portfolio management teams that were ingesting different forms of alternative data, such as transaction and web-surfing information, and thinking about how to use cell-phone location data for their investment decision-making processes.
“What we see on our end, in the raw data, is we may get a location when someone is up in the morning, getting ready for work, on the commute to work, at the office sitting in a conference room, at lunch, on the way home stopping at a grocery store—you get the idea,” Collins says. “We get a really rich sort of sequence of locations visited because we have all of this passive collection from many different sources.”
Thasos is far from the only company to recognize the potentially rich yield from this data—that is, if it can only be properly harvested.
One Billion Eyes
There are over one billion smart phones in the world. Most modern devices have the capability to geo-locate and generate data about users’ foot traffic, information that has become a category of alternative data used by a small but growing number of hedge funds.
The most common way such data is collected is by asking consumers for their location through mobile applications such as a navigation, weather, shopping and/or social media. The apps generally obtain location information in a passive way, meaning even when the consumer is not using it, they will automatically grab the latitude and longitude that represents the position of the phone at any given time.
Vendors have been quick to catch on. Last year, in March, Thasos used the knowledge it had gained from managing its portfolio to launch a real-time data series product, Streams. As the name suggests, it tracks a stream of foot traffic to a point of interest, and offers information on a variety of performance metrics ranging from areas such as employee hours worked on an assembly line and patient counts in hospitals through to foot traffic at stores, restaurants and malls. Thasos’ clients include hedge funds, as well as commercial real estate firms and a handful of retailers.
Advan Research is another vendor working with location data. Its CEO, Yiannis Tsiounis, predicts that in the next year or two, 1,000 hedge funds will incorporate these datasets into their strategies. He describes it as a new frontier for providing alpha.
“The financial industry has to go into some alternative data in order to survive,” says Tsiounis. “It is a survival issue. If you don’t do it, you might get lucky and have a good quarter, a good year, but how can you consistently over-perform a passive index if you don’t have some additional information advantage? So the industry has to go to alternative data, and I would say in the last 12 to 18 months, they have actually realized that.”
Right Tool, Right Job
Another use for location data is as an independent check on existing investment theses that may be based on other datasets. No one type of alternative data is perfect, and studying different types can help reduce risk. Thasos’ Collins cites several examples of hedge funds that, based on mobile phone location data trends that were diverging from transaction data, decided to pull out of a position in which they had already done quite well.
An inevitable question for hedge funds to ask is if mobile geolocational data is the right type of dataset to be looking at. The range of alternative datasets is in the thousands, with new types emerging all the time, and particularly through partnerships between data providers and specialist market participants. One such example occurred when Marex Spectron, a commodities broker, recently announced a joint venture with Earth-i, to develop and distribute a range of analytics tools. They will initially focus on the copper market, combining Earth-i’s satellite imagery and video analytics with Marex Spectron’s datasets.
According to Guy Wolf, Marex Spectron’s global head of market analytics, modern technology is capable of taking images at multiple angles, which permits the creation of three dimensional images of objects on the ground, as opposed to two-dimensional. Any changes in a mining pit, for instance, will correlate to the volume of earth removed, or it could be used for measuring the size of stockpiles.
As with any type of data, there are challenges with satellite imagery. “The biggest challenge in satellite data is, frankly, cloud cover. The advantage of Earth-i’s constellation is that it actually allows for multiple pass-overs every day, whereas a lot of satellite structures allow for only one potential image opportunity per day.”
Is there a particular reason they are not looking at mobile geolocational data? “I struggle to see what the applications would be within the metals market, to be honest,” says Wolf. “Ultimately, we are only interested in data that can provide insights into what goes on in the metals derivatives markets, and with geolocation data I struggle to see what the use-case could be.”
While it may be true that particular types of alternative data may be more applicable to a certain industry, a hedge fund investor who spoke with Waters says he figured out how to use mobile geolocation data under certain circumstances.
“For metals and mining, if you wanted, you could measure the number of trucks coming in and out of a copper yard,” he says. “It is useful; you just have to be smart enough to figure out how to use it. That was kind of my value add. When I figured out how you correlate these alternative datasets to things that matter or interest. Some things are more useful in some places and less useful in others. Like credit card data is not going to be useful to help you figure out global copper stuff, but you can definitely use geolocation data productively.”
But even though service providers and data brokers are stepping into this new field, much of the footwork to gain any actionable insight rests squarely with the person on the trading desk.
This was the experience of one US-based hedge fund analyst, who asked not to be named when he spoke to Waters. When he joined the fund, his employers were buying some of this data, but they weren’t really using it as they didn’t have anyone on staff with the right skills or desire to do it. It was a more traditional long–short equity fund where they would rely on management calls, reading transcripts or having people go visit actual stores and malls to try and understand how a company was performing.
His employers didn’t have a data scientist, so he spent about 20 percent of his time doing the grunt work required to analyze these datasets, and the remaining 80 percent of his time turning the data into actionable strategies. “I knew some of this stuff was there, it was being unused, and I have the right math and stats background to actually use this stuff and connect it with traditional investing,” he says.
According to this analyst, it is getting more difficult to make money solely using traditional data. “Most hedge funds that rely on traditional data—meaning like Capital IQ or Bloomberg, or whatever—they are no longer generating returns. I was able to return a 20 percent market-neutral alpha by using alternative data,” he says.
He was buying data from firms like Thasos, who pre-process it. But he found the data in itself was not useful. “The raw data is hundreds of gigabytes of data that you can buy in raw form,” he says. “You need a huge team of data scientists and cleaners to clean up the data; that is step one and that is what places like Thasos do. After that, you have to realize, now that you have cleaned the data, how do you turn it into something you can use to actually make money or make it investible? That second piece I was doing.”
Finding this blend of scientist and trader, however, is harder than it seems.
A lot of people buy such data; the challenge is having somebody who can understand it. “Even in the traditional hedge fund world, what differentiates a good versus bad hedge fund is not whether they are buying Bloomberg data or not; everybody has it. It’s the people who learn to extract more value from that same source of information versus their peers,” says the analyst.
Most traditional investors, whether mutual funds or hedge funds, need someone who has both a math or statistics background, as well as an understanding of how a business works. According to the analyst, most places have one or the other. The quant funds have people who are very good at the math, but don’t understand the business side. The inverse is true for traditional asset managers that typically lack qualified personnel to analyze the data.
Most hedge funds buy processed data from vendors like Thasos and Advan. But if a fund feels it has a very strong data science team and technical infrastructure, it may be able to glean additional insights from the data that others cannot, according to Octavio Marenzi, CEO of consultancy Opimas, and the author of a recent report on generating alpha from mobile geolocational data.
However, the number of people who can really do that is very small. Marenzi says he is only aware of one fund, Two Sigma, that is buying the raw data and doing the analytics in-house. Two Sigma did not respond to a request for comment.
“Most others, in the top-tier funds, might buy the raw data, but then they might buy the normalization algorithms from somebody else, and then tinker around themselves with it to try and improve the data quality. But I think the vast majority of people are not going to have the skills or the resources to do that and are simply going to buy the finished analytics from these providers,” Marenzi says.
Even if such a capability is found, however, the data can just as often be a wild goose chase rather than a golden egg.
Data Wrangling
Mobile geolocation data has problems with accuracy. For example, to know how many people were inside a retail chain you must be aware of the exact polygons and shapes of the shops. “It becomes a bit difficult because there might be someone walking by the outside of an H&M shop on a busy street and that could look like someone who is in a shop, even though they are not, and that becomes even worse in smaller locations like Starbucks,” says Marenzi.
That can then also be skewed by things like a major road that goes right by a location, with the risk of picking up all that traffic that is just driving by. “You need very accurate data to be able to do that,” says Marenzi.
Another difficulty Marenzi notes is that mobile apps can wary widely from day to day, from month to month, in terms of how many users they have. According to Marenzi the data currently is not nearly as clean as users would like it to be. “You can get some interesting information out of it but there is so much work that needs to be done to sort of normalize the data and get a clean signal,” he says.
Another general concern with mobile geolocation data is privacy. Tsiounis of Advan Research has a PhD in cryptography and a bachelors degree in mathematics. “My PhD was actually in privacy and anonymity. So I did anonymous electronic cash. I was building algorithms specifically to protect privacy,” he says.
Tsiounis says geolocation is one of, if not the most transparent dataset in terms of privacy. “You explicitly are told that this data is going to be used and resold and you get permission. Compare that to point-of-sale data: I swipe my card to buy something, somebody takes that information and sells it. They didn’t ask me,” he says.
According to the report by Opimas, the market for mobile geolocation data is expected to exceed $250 million by 2020. Some of the problems with data accuracy could start changing with the 5G connectivity standard, which is supposed to be position-accurate down to about one meter, and will give almost continuous location updates. The problem with 5G when it first really comes live, notes Opimas’ Marenzi, is that there won’t be historical data to compare it to. Investors will have to wait for a year or two to have enough historical data to make comparisons and figure them out—that is, if they can stomach the requirements for doing so.
The hedge fund analyst, who recently left his job, is currently in the process of starting his own fund to focus exclusively on using alternative data to generate alpha. But will his old firm still be using such data?
Probably not, he says, because he doesn’t imagine his former employers will continue to use the data now that he’s left. After all, he says, they lack the interest and skill set to actually do this without him. Time will tell who’s right.
Only users who have a paid subscription or are part of a corporate subscription are able to print or copy content.
To access these options, along with all other subscription benefits, please contact info@waterstechnology.com or view our subscription options here: http://subscriptions.waterstechnology.com/subscribe
You are currently unable to print this content. Please contact info@waterstechnology.com to find out more.
You are currently unable to copy this content. Please contact info@waterstechnology.com to find out more.
Copyright Infopro Digital Limited. All rights reserved.
As outlined in our terms and conditions, https://www.infopro-digital.com/terms-and-conditions/subscriptions/ (point 2.4), printing is limited to a single copy.
If you would like to purchase additional rights please email info@waterstechnology.com
Copyright Infopro Digital Limited. All rights reserved.
You may share this content using our article tools. As outlined in our terms and conditions, https://www.infopro-digital.com/terms-and-conditions/subscriptions/ (clause 2.4), an Authorised User may only make one copy of the materials for their own personal use. You must also comply with the restrictions in clause 2.5.
If you would like to purchase additional rights please email info@waterstechnology.com
More on Emerging Technologies
Waters Wavelength Ep. 300: Reflecting on humble beginnings
It is our 300th episode! Tony and Shen reflect on how it all started.
An inside look: How AI powered innovation in the capital markets in 2024
From generative AI and machine learning to more classical forms of AI, banks, asset managers, exchanges, and vendors looked to large language models, co-pilots, and other tools to drive analytics.
Asset manager Saratoga uses AI to accelerate Ridgeline rollout
The tech provider’s AI assistant helps clients summarize research, client interactions, report generation, as well as interact with the Ridgeline platform.
LSEG rolls out AI-driven collaboration tool, preps Excel tie-in
Nej D’Jelal tells WatersTechnology that the rollout took longer than expected, but more is to come in 2025.
The Waters Cooler: ’Tis the Season!
Everyone is burned out and tired and wants to just chillax in the warm watching some Securities and Exchange Commission videos on YouTube. No? Just me?
It’s just semantics: The web standard that could replace the identifiers you love to hate
Data ontologists say that the IRI, a cousin of the humble URL, could put the various wars over identity resolution to bed—for good.
T. Rowe Price’s Tasitsiomi on the pitfalls of data and the allures of AI
The asset manager’s head of AI and investments data science gets candid on the hype around generative AI and data transparency.
As vulnerability patching gets overwhelming, it’s no-code’s time to shine
Waters Wrap: A large US bank is going all in on a no-code provider in an effort to move away from its Java stack. The bank’s CIO tells Anthony they expect more CIOs to follow this dev movement.