Nasdaq's Lessons in Machine Learning Yield New Surveillance Tool
The offering, which took more than a year to build, combines deep, transfer and human-in-the-loop loop learning to find patterns.
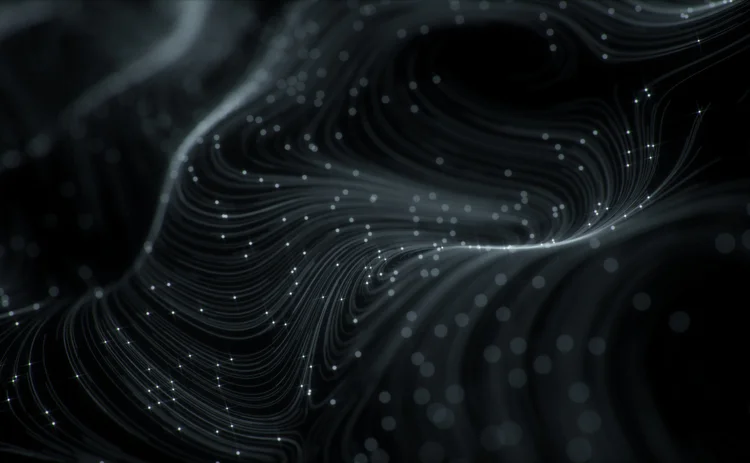
Yesterday, Nasdaq announced the launch of its new AI-powered market surveillance tools for finding patterns of abusive behavior. Underpinning the new technology are subsets of machine learning (ML): deep learning, for analyzing extremely complex relationships and understanding hidden insights within massive data sets; transfer learning, which involves training new models based on older ones to allow scale and save time; and human-in-the-loop learning, which requires human interaction to weed out noise from signal, especially where it’s not cut and dry.
If we could reduce something like spoofing to a signal, and the shape of the signal will have some consistency across many different asset classes, it made us very hopeful that techniques like transfer learning would be very successful for looking at these different patterns from US equities to, for example, a commodities market.
Tony Sio, Nasdaq
Currently, the tool covers only US equities, but the stock exchange has already run some trials on fixed income and with other exchanges, which have shown promise, Tony Sio, head of marketplace regulatory technology at Nasdaq, tells WatersTechnology. The project had been in the works for more than a year and was born from the company’s initial forays into machine learning, which were focused on alert scoring in the Nordics.
“We had very positive results; we had a lot of learnings, and we deployed that,” Sio says. “Based on that experience, we felt we could do a lot more. So after that, we really pushed the deep learning project against the low-level data coming from our trading system to detect patterns of abuse.”
But those first explorations into machine and deep learning bumped into another project. A separate team was working on how to create a visual picture of spoofing, and the missing ingredient also lay somewhere in machine learning.
“We called it the signature of spoofing,” Sio says. “We showed it to a lot different people, and people said, ‘Yes, I look at this picture, and I see it.’ And that coincided with some of the ML technologies out there, which are really about taking those visual patterns and being able to find them in the data. It’s two separate initiatives starting to overlap.”
The teams distilled all the data—which was extracted from Nasdaq’s trade engine—down to a single chart with a few different signals, such as ones indicating trading behavior and the volume of the order book at different price sets. They showed the charting method to surveillance professionals down the line of asset classes—equities, commodities, futures and more. What they found was the signal looked similar across all of them.
“If we could reduce something like spoofing to a signal, and the shape of the signal will have some consistency across many different asset classes, it made us very hopeful that techniques like transfer learning would be very successful for looking at these different patterns from US equities to, for example, a commodities market,” Sio says.
Human-in-the-loop training adds another methodical layer to surveillance. By using actual examples from the 750,000+ alerts that Nasdaq’s US surveillance team sifts through per year, the human and machine-powered model goes beyond just deciding whether an alert is interesting or not. It can decide, for example, that an alert is of interest, but only for a certain time frame, or that a certain day brought interesting activity, but only in the afternoon.
But it’s the transfer learning that is most core to the project’s mission. For Sio, ensuring market integrity has to be a collaborative effort. He sees opportunity for Nasdaq’s surveillance technology customers, other exchanges, and, particularly, smaller marketplaces.
“One really interesting concept is the concept of transferring those learnings from one marketplace to another and potentially back again. I think that’s a hugely-powerful concept,” Sio says. “One of the things we heard from smaller marketplaces is that they have less examples of many of these abusive behaviors. It’s just quite a lot more work for them to train models using the small examples they have.”
Along the way, Sio says he and the teams learned some vitally-important things. Perhaps the key one, though, was they had to make sure they had the interaction with users onboard, and a way to imbue the machine-learning tools with the nuanced ways the surveillance team thinks, such as their abilities to predict when certain behaviors are more or less likely.
“The problem in surveillance is one where you’re trying to find needles in the haystack,” he says. “There are billions of messages coming in every day. There are patterns and the patterns look different or change over time, and you’re just trying to find those behaviors.”
Further reading
Only users who have a paid subscription or are part of a corporate subscription are able to print or copy content.
To access these options, along with all other subscription benefits, please contact info@waterstechnology.com or view our subscription options here: http://subscriptions.waterstechnology.com/subscribe
You are currently unable to print this content. Please contact info@waterstechnology.com to find out more.
You are currently unable to copy this content. Please contact info@waterstechnology.com to find out more.
Copyright Infopro Digital Limited. All rights reserved.
As outlined in our terms and conditions, https://www.infopro-digital.com/terms-and-conditions/subscriptions/ (point 2.4), printing is limited to a single copy.
If you would like to purchase additional rights please email info@waterstechnology.com
Copyright Infopro Digital Limited. All rights reserved.
You may share this content using our article tools. As outlined in our terms and conditions, https://www.infopro-digital.com/terms-and-conditions/subscriptions/ (clause 2.4), an Authorised User may only make one copy of the materials for their own personal use. You must also comply with the restrictions in clause 2.5.
If you would like to purchase additional rights please email info@waterstechnology.com
More on Regulation
Removal of Chevron spells t-r-o-u-b-l-e for the C-A-T
Citadel Securities and the American Securities Association are suing the SEC to limit the Consolidated Audit Trail, and their case may be aided by the removal of a key piece of the agency’s legislative power earlier this year.
BlackRock, BNY see T+1 success in industry collaboration, old frameworks
Industry testing and lessons from the last settlement change from T+3 to T+2 were some of the components that made the May transition run smoothly.
How ‘Bond gadgets’ make tackling data easier for regulators and traders
The IMD Wrap: Everyone loves the hype around AI, especially financial firms. And now, even regulators are getting in on the act. But first... “The name’s Bond; J-AI-mes Bond”
Can the EU and UK reach T+1 together?
Prompted by the North American migration, both jurisdictions are drawing up guidelines for reaching next-day settlement.
Waters Wavelength Ep. 293: Reference Data Drama
Tony and Reb discuss the Financial Data Transparency Act's proposed rules around identifiers and the industry reaction.
Clearing houses fear being classified as DORA third parties
As the 2025 deadline looms, CCP and exchange members are seeking risk information that’s usually deemed confidential.
Industry not sold on FIGI mandate for US reg reporting
Banks’ and asset managers’ tortured relationship with Cusip numbers remains tortured, as they tell regulators to keep the taxonomy in play.
T+1 shift sees out-of-hours human resourcing costs spike by as much as 20%
New research finds that trading firms are experiencing increased labor costs—which could be a boon for outsourced trading.