Bot’s job? Quants question AI’s model validation powers
But supervisors cautiously welcome next-gen model risk management

Need to know
- Model validation is crucial to ensuring that banks’ risk models are fit for purpose—a labor-intensive task overseen by quants and, until now, mostly carried out by humans.
- But now, providers of solutions that deploy artificial intelligence for model validation say tens of banks are interested and some are already using such tools.
- Meanwhile, regulators in Europe and the US have expressed their support for the use of AI—or machine learning—for some aspects of model validation.
- But some quants are wary of using one form of AI to police another—and caution against replacing human brainpower in model validation—especially in areas of high criticality.
Can bots police bots? It’s a conundrum at the center of many a sci-fi thriller. But now the real-world question is whether bank bots can validate their own models—or perform the crucial job of ensuring risk management models are fit for purpose. Some quants don’t think they can.
While banks already deploy artificial intelligence (AI) for many tasks—such as recognizing patterns in financial data, calculating risk sensitivities or finding the optimal execution for a trade—when it comes to model validation, those tasked with its oversight are not in favor.
“Model hacking is not an easy job, and needs creativity,” argues Agus Sudjianto, head of corporate model risk at Wells Fargo. He says model validation is a destruction-testing exercise in hacking a model, and believes human brainpower is critical.
It’s a common view among his quant colleagues that AI—or machine learning—can’t equal or better human intuition in the field. Five quants interviewed for this story say they are resistant to the idea of AI replacing the assessments they carry out.
But such sentiments do not deter tech giant IBM, which is making “a big bet” on introducing AI to model validation. The firm says “tens of banks”—particularly tier-one banks—are interested in its solution. Some are already in production, while others are working to deploy, in both North America and Europe.
“You tend to start to see these types of things adopted at the top first, and then it sort of trickles down to tier two, tier three-type banks,” says Marc Cassagnol, product manager in regtech, data and AI at IBM Software.
Jos Gheerardyn also believes tens of banks globally have this type of solution in production. He is chief executive officer of Yields.io, a fintech platform that has offered AI for real-time model testing and validation since 2017.
It’s here that AI can help most with assessing data quality and identifying anomalies in the underlying distribution of data, says Gheerardyn. It can also contribute to building challenger models and risk quantification to measure how uncertain the outcome of a particular model is.
Validating fraud detection models is another use case to which AI is well suited, says Cassagnol, who claims IBM’s offering has “picked up speed” in the past year: “It’s a big bet that we are hoping works out.” He says a few dozen developers work on IBM’s product, with hundreds of salespeople around the world.
Banks typically need a critical mass of models in their inventory for the implementation to make economic sense, says Gheerardyn, and their prime driver is a need to cut the costs of model validation.
One large emerging markets bank uses the solution to reduce the time it takes to validate models—from several days or more down to less than 30 minutes. It uses the solution for what it deems low-criticality models, achieving a 60% reduction in manual activities associated with model validation. Models rated medium risk or higher will always get human review, but lower risk models are validated without any human interaction.
Regulators are not averse to the use of AI in model validation either. Some say it can be used to speed things up, create challenger models and benchmarks. The European Banking Authority (EBA) is “technologically neutral”, says Lars Overby, its head of credit, market and operational risk policy.
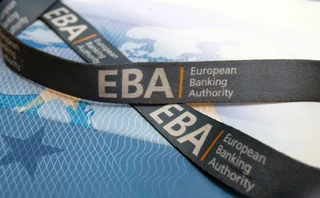
“You can use machine learning techniques to help improve the models, provide challenger models to what is already in place and similar applications—all of this is something that we’re very positive towards,” he says.
“For instance, a neural network application on credit risk data could be useful for identifying the correct grouping for bands used for probability of default groupings.”
But regulators also don’t want humans removed from the equation. “You still have the challenge of the methodological choice and the optimization that needs to be done by experts,” says Overby.
Validation tasks that AI is less helpful with are qualitative in nature, such as identifying the level of model documentation, assessing business cases and estimating how complex code has been organized.
And an important pitfall to avoid when using AI in model validation is to ensure it doesn’t introduce model risk where it should be detected.
“We’re kind of getting a bit meta here, talking about a model to assess other models. So there’s a different kind of risk involved there,” says IBM’s Cassagnol.
“When your model performs poorly, there [are] certainly bad consequences.”
Manhandling the models
In addition to the standard testing of catching mistakes that model developers make, validation must catch a model’s vulnerability to being wrong in different situations. As well as ‘bumping’ variables to check whether a model holds in certain scenarios, the model validator has to think under which operating conditions the model may not work. Bumping stresses the parameters of a model to check how it reacts and verify if it holds when market conditions change.
For Wells Fargo, model hacking includes searching an area where a model will be the weakest, testing for robustness with input changes, finding areas where the decision of the model has higher uncertainty and then asking: “If the world changes, does the model still work?”
“While AI may be able to help with that, as well as automate outcome analysis to catch errors in logic, it cannot prove the conceptual soundness required in model validation,” says Sudjianto, who has published work on making machine learning interpretable and explainable. “That involves economic theory, and an AI model will not be able to do that.”
One quant—also skeptical about the use of machine learning approaches for areas where analytical techniques would normally be used—agrees the technology may have a role in positing challenger models. He thinks, for example, it could make sense to compare the performance of pricing models for structured products with a high complexity of cashflow depending on econometric factor projections with mock models set up by using modern machine learning techniques.
![]()
You can use machine learning techniques to help improve the models, provide challenger models to what is already in place and similar applications—all of this is something that we’re very positive towards
Lars Overby, European Banking Authority
This could provide a benchmark to assess the quality of data being used, to see what is closest to the noise-signal boundary, and the maximum information that can be extracted from historical data. A downside, he adds, is that if a model has no performance issues, it could take a lot of time and effort to design the AI algorithm to challenge it. And if there are performance issues, his team could anyway use the occasion to redesign the primary model itself, working closely with traders who may likely have some insights about any specific issues.
The automated model validation solution at the large emerging markets bank consists of an engine that takes the model and runs similarity tests, as well as classification and regression metrics, then compares the model with a baseline. It detects a range of problems, including those related to database overfitting and accuracy. If all is well, the model is put into production without the need for human oversight.
An example of such a model would be one that calculates the probability of a fraudulent payment. Other use cases could include credit risk models that estimate probability of default, exposure at default and loss given default.
Regulatory reception
Regulators are certainly not cold to the concept of using machine learning in model validation—albeit with some caveats—and want to see no resulting reduction in model risk management.
Last year, the EBA published a paper in which it advised that in the case of internal ratings-based models, machine learning can provide added value, but should also be capable of being interpreted.
“It’s important that the results are interpretable—the explainability of results,” says Overby.
There is otherwise a danger of developing ‘black-box’ models, introducing the risk that decisions are delegated to an algorithm that is not fully understood. The problem could be compounded if turnover in model-building staff were to see relevant expertise moving from the institution—or if work on models were to be outsourced to third-party providers. Banks cannot outsource responsibility for model risk, and must conduct thorough internal audits on their model risk management processes, says Overby.
If a model is used for internal purposes, such as a credit risk model for internal pricing, or in fraud detection—rather than for setting capital requirements—less strict regulatory requirements apply, adds Overby.
When a fraud model is wrong, we can lose a lot of money
Agus Sudjianto, Wells Fargo
In its paper, the EBA seeks more clarity on how model validation is performed using AI, and is currently deliberating next steps to take that consultation forward.
In the US, banks putting AI to work in model validation need to comply with supervisory guidance SR 11-7 on model risk management. First published in 2011, the guidance applies to banks supervised by the US Federal Reserve Board and the Office of the Comptroller of the Currency.
US regulators characterize themselves as open to innovation, provided it is done responsibly. They are already pushing banks to demystify their use of machine learning. But parts of SR 11-7 require inspection and effective challenge by appropriate parties—and it’s questionable whether this requirement can be automated during validation.
Essential to SR 11-7 are, at a minimum, annual reviews of all models, covering everything from their ‘conceptual soundness’ to ongoing monitoring and outcomes analysis. For validation purposes, it requires a conceptual soundness assessment to be carried out objectively—and not by those involved in model development—a process that an AI program is unlikely to be able to carry out. Banks may only defer validation in exceptional circumstances if there is an immediate need, as happened in 2021 with respect to Bank Secrecy Act/Anti-Money Laundering compliance, in order to quickly adapt to an evolving threat environment.
US regulators do see a role for AI-like technology in general time-saving tasks that would otherwise burden humans: automatic alerts for when model performance deteriorates, automated documentation templates, automated handoff in the validation process and relational databases where key validation information is stored.
The level of materiality in a model should drive the intensity and frequency of model risk management activities, according to SR 11-7. For models of lower materiality, firms can use a lighter touch, spend less time reviewing and revalidating those models, and could have more junior people assess them.
But regulators do not draw a line in the sand to specify which models are suitable for automated validation. They generally envisage human participation in model risk management practices—especially in model-validation practices, where they see AI as complementing human brainpower—and the degree of human input into validation should be determined by a firm’s internal audit function.
Healthy skepticism
Some quants question the role of AI in their patch, full stop.
A senior quantitative analyst at a large German bank says: “There’s actually no task in our department which is done by something like AI.” The bank does use new procedures in Python for name-matching—translating corporate names into vectors, and matching them by calculating the angle between those vectors—“but to the extent that we could call it AI, no, that’s not the case”.
The models he validates in the field of credit risk take on average two months: “The whole validation process is not only about running code and checking data. It’s a very human process because a good validation really thrives on a discussion that you have with the model owner about model assumptions or the model approach itself.”
These discussions may take in big macro elements, such as environmental factors, or changes in the economy, says the quant: “The disadvantage of code or AI is that it doesn’t have a very comprehensive view on things.”
With regard to model validation, he adds: “I’ve never heard about anyone applying AI, because the downside is that if you use AI to validate the model, then maybe another validation task arises that has to validate the AI.”
A second quant says the time-consuming aspect of model validation is actually executing the models. Colin Turfus, a senior quant analyst with experience at several investment banks, says: “Insofar as model validation is usually defined by regulators as requiring a repeat of the test under new sets of market conditions, essentially, you have to run the models again. And the bottleneck is how long it takes to actually run your model. So, if your model is a Monte Carlo simulation, and it takes you 10 minutes to calculate a price, you cannot do model validation in less than the sum total of the CPU [central processing unit] time it takes to run these 10-minute tests.”
Turfus also believes that human input is essential: “Model validation, as I understand it—properly done—means that an experienced quant looks at the model, and considers the things that might be wrong. And designs tests which will try to stress that model.”
He thinks a machine learning approach might be of assistance by making use of historical data to generate credible problematic future scenarios. At the moment, however, regulators tend to prescribe stress scenarios that replicate past shocks in a more deterministic way. He thinks this can be “a poor way of stress-testing a model because you’re guaranteed always to be looking at the problems that we had in the past, leaving us open to being blindsided by the new ones”.
In what Turfus calls a “gold-standard” validation process, the output of a pricing model should be compared with a benchmark, for example. Generally, model validation teams don’t have the resources to create challenger models for every model, as they take a long time to build. So, instead, consistency checks are substituted—or other ways of indirectly checking a model is working. AI may be able to assist validation in this area, he says.
![]()
If your model is a Monte Carlo simulation, and it takes you 10 minutes to calculate a price, you cannot do model validation in less than the sum total of the CPU time it takes to run these 10-minute tests
Colin Turfus, senior quant analyst
IBM’s Cassagnol says an AI validation model should be subject to the same governance processes as every other model, and its ongoing performance monitored.
That includes “checking for all of the sort of quality, fairness and drift issues that happen with AI models, and making sure that if any of those go beyond the threshold, you stop the model until you figure [out] what’s going on”.
All models at the large emerging markets bank are validated, including those used in AI. The bank follows global guidelines and best practices, and while its national regulator doesn’t prescribe model rules, it obeys general risk governance principles.
Gheerardyn at Yields.io concedes that introducing machine learning techniques will introduce additional model risk, but says that new techniques in model risk management are not employed in the highest tiers of heavily regulated models. Yields.io finds a sweet spot in “middle-tier-type models” that have less materiality. Conversely, models in the lowest tier of materiality may lack quantitative aspects that would benefit from automated validation.
Challenger models could be validated by creating yet another challenger model, he says: “But, at some point, you will have to stop.”
Yields.io has developed multiple AI explainability techniques on its platform to create benchmark models. But as it is mostly employing machine learning techniques to create benchmarks or challenger models, the machine learning models are not themselves running in production at banks.
“In that case, the need for explainability on the machine learning side is a second-order thing. You often want to have explainability implemented properly in the production model. While in the challenger model, that is obviously important, it’s a second-order risk related to the model that is being validated,” says Gheerardyn.
But not everyone is convinced.
Wells Fargo’s Sudjianto says his bank also risk-tiers its models, from one to four, with tier one being the most material, according to the impact on the business if the model is wrong. But he would not necessarily deem a fraud detection model, for example, to be of low criticality.
“When a fraud model is wrong, we can lose a lot of money.”
Only users who have a paid subscription or are part of a corporate subscription are able to print or copy content.
To access these options, along with all other subscription benefits, please contact info@waterstechnology.com or view our subscription options here: http://subscriptions.waterstechnology.com/subscribe
You are currently unable to print this content. Please contact info@waterstechnology.com to find out more.
You are currently unable to copy this content. Please contact info@waterstechnology.com to find out more.
Copyright Infopro Digital Limited. All rights reserved.
As outlined in our terms and conditions, https://www.infopro-digital.com/terms-and-conditions/subscriptions/ (point 2.4), printing is limited to a single copy.
If you would like to purchase additional rights please email info@waterstechnology.com
Copyright Infopro Digital Limited. All rights reserved.
You may share this content using our article tools. As outlined in our terms and conditions, https://www.infopro-digital.com/terms-and-conditions/subscriptions/ (clause 2.4), an Authorised User may only make one copy of the materials for their own personal use. You must also comply with the restrictions in clause 2.5.
If you would like to purchase additional rights please email info@waterstechnology.com
More on Emerging Technologies
Waters Wavelength Ep. 295: Vision57’s Steve Grob
Steve Grob joins the podcast to discuss all things interoperability, AI, and the future of the OMS.
S&P debuts GenAI ‘Document Intelligence’ for Capital IQ
The new tool provides summaries of lengthy text-based documents such as filings and earnings transcripts and allows users to query the documents with a ChatGPT-style interface.
The Waters Cooler: Are times really a-changin?
New thinking around buy-build? Changing tides in after-hours trading? Trump is back? Lots to get to.
A tech revolution in an old-school industry: FX
FX is in a state of transition, as asset managers and financial firms explore modernizing their operating processes. But manual processes persist. MillTechFX’s Eric Huttman makes the case for doubling down on new technology and embracing automation to increase operational efficiency in FX.
Waters Wavelength Ep. 294: Grasshopper’s James Leong
James Leong, CEO of Grasshopper, a proprietary trading firm based in Singapore, joins to discuss market reforms.
The Waters Cooler: Big Tech, big fines, big tunes
Amazon stumbles on genAI, Google gets fined more money than ever, and Eliot weighs in on the best James Bond film debate.
AI set to overhaul market data landscape by 2029, new study finds
A new report by Burton-Taylor says the intersection of advanced AI and market data has big implications for analytics, delivery, licensing, and more.
New Bloomberg study finds demand for election-related alt data
In a survey conducted with Coalition Greenwich, the data giant revealed a strong desire among asset managers, economists and analysts for more alternative data from the burgeoning prediction markets.